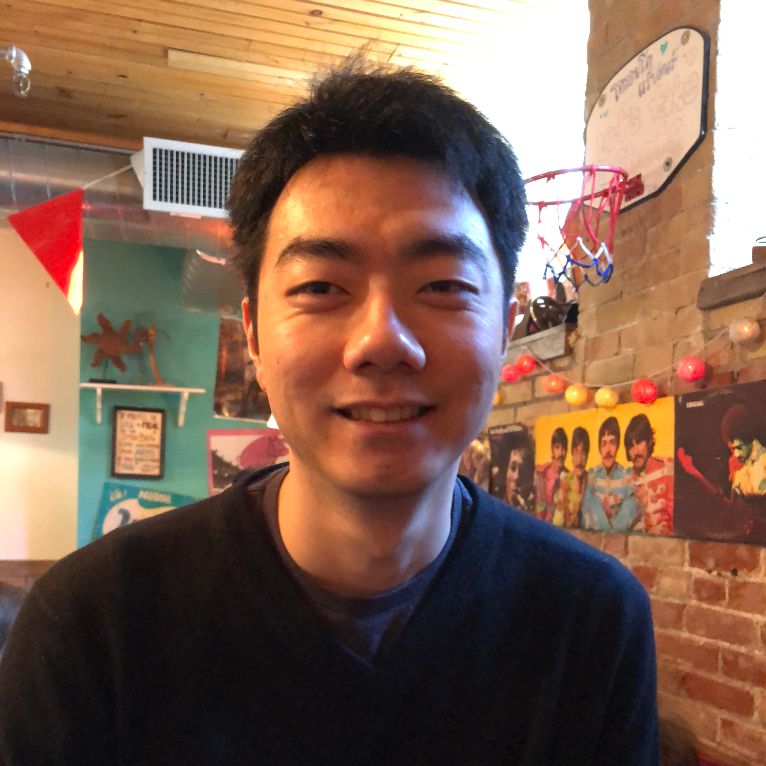
I'm a Research Scientist at Meta Fundamental AI Research (FAIR) team in New York. My research is on building simplified abstractions of the world through the lens of dynamical systems and flows.
Lately, I've been exploring the use of stochastic control theory for large-scale generative modeling [Adjoint Matching], as well as constructing discrete generative models through continuous-time Markov chains [Discrete Flow Matching]. Our methods such as [Flow Matching] have been applied successfully for foundation models of video and audio [Movie Gen].
CV | Github | Twitter | Google Scholar | rtqichen@meta.com
Talk Slides
- Stochastic Control for Large Scale Reward-Driven Generative Modeling ICLR 2025 Workshop on Deep Generative Model in Machine Learning: Theory, Principle and Efficacy 2025 slides | website
- Flow Matching Tutorial NeurIPS 2024 2024 slides | website
- The Flow Matching Recipe for Generative Modeling: from continuous to discrete ICML 2024 Workshop on Structured Probabilistic Inference & Generative Modeling 2024 slides | website
- Flow Matching on General Geometries [15min version] ICLR 2024 Oral Presentation 2024 slides | website
- Discovering Latent Dynamics of the World: A Simulation-Free Perspective Vanderbilt Machine Learning Seminar Series 2024 slides | website
- Flow Matching on General Geometries Learning on Graphs Conference New York Meetup 2024 slides | website
- Generative Flows: Beyond Distribution Matching Flatiron Institute Workshop on Topics of Measure Transport 2023 slides | website
- Simulation-free generative modeling with neural ODEs ICIAM 2023 Theoretical and computational advances in measure transport 2023 slides | website
- Simulation-free generative modeling with neural ODEs AFOSR Topics at the Intersection of Deep Learning and Computational Nonlinear Control 2023 slides | website